By Angelo Centonza, 3GPP Working Group RAN3 Vice Chair
In September 2020, RAN plenary approved a new study to investigate support for Artificial Intelligence and Machine Learning (AI/ML) in 5G RAN architectures. It explored a functional framework for RAN intelligence and identified use cases, based on the current 5G RAN architecture, where the application of AI/ML techniques could bring substantial benefits.
The RAN3 study, concluded at plenary (RAN#95-e) in March 2022, was motivated by the strong interest shown by the 3GPP community in pushing RAN automation towards new targets, with the aim of achieving network optimisation decisions in multi-variable scenarios for which classic, “rule-based” techniques would not be equally effective.
The approval of TR 37.817 - Study on enhancement for data collection for NR and ENDC marked a symbolic point in the history of 3GPP: standardisation moved from measurement-fuelled processes to methods that make use of predictions. Not only that, but such methods would also be able to predict events within an identified event class, that were seldom - or perhaps never - recorded before.
This study was the first of its kind and its outcomes are influencing work in similar activities of other 3GPP WGs, such as RAN1, SA5, and SA3.
3 main use cases investigated
In order to focus the effort on tangible solutions and to ensure convergence to well-defined guidelines for normative work, RAN3 identified three main use cases for which AI/ML based solutions would be investigated:
- Network Energy Saving: where the energy consumption improvements for the whole radio access network may be achieved by actions such as traffic offloading, coverage modification and cell deactivation.
- Load Balancing: where the objective is to distribute load effectively among cells or areas of cells in a multi-frequency/multi-RAT deployment to improve network performance based on load predictions.
- Mobility Optimization: where satisfactory network performance during mobility events is preserved while optimal mobility targets are selected based on predictions of how UEs may be served.
One of the first steps taken by RAN3 was to identify key principles on the basis of which technical solutions for each use case could be developed. The following are some of the most relevant principles agreed:
AI/ML algorithms and models are implementation specific and out of standardisation scope. | This principle ensures free model selection and the establishment of a framework that fosters competition. |
Solutions development should focus on the enabled AI/ML functionality and the definition of the corresponding types of inputs/outputs. | Namely, the main scope of the study includes the definition of the corresponding types of inputs/outputs and on determining how to transfer needed information among AI/ML functions, so to enable the desired AI/ML functionality. |
Location of AI/ML functionalities within the current RAN architecture depends on deployment and on the specific use cases. | Namely, there would be flexibility on where AI/ML functionalities reside, depending on the use case and the solution selected. |
Model Training and Model Inference functions should be able to request data needed for AI/ML purposes, if needed. | Namely, information needed to run an AI/ML based process may be provided on a subscription basis. |
User data privacy and anonymisation should be respected during AI/ML operation. | It remains to be analysed whether specific solutions are needed. |
Introducing AI and ML while maintaining the current 5G architecture was a challenge that RAN3 addressed very effectively. In order to facilitate such a task, RAN3 defined a functional framework aiming at providing guidance on how different AI/ML functions interwork, this is shown in the figure below:
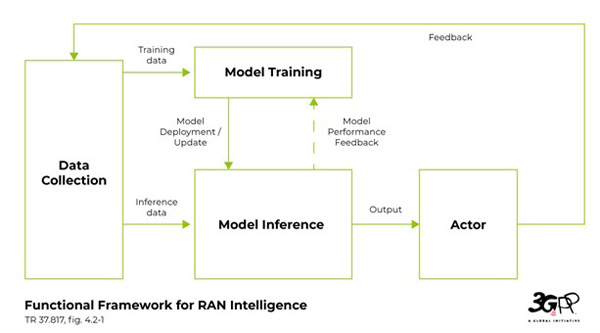
Deployment options
On the basis of the principles and framework above, RAN3 developed different deployment options for the AI/ML functions:
- AI/ML Model Training is located in the OAM and AI/ML Model Inference is located in the gNB (gNB-CU for split RAN).
- AI/ML Model Training and AI/ML Model Inference are both located in the gNB (gNB-CU for split RAN).
For every use case, RAN3 identified sets of AI/ML inputs, outputs and feedback information that were considered most relevant to carry out and evaluate AI/ML based processes.
A variety of input information was described. Inputs may be generated by different entities such as UEs, neighbouring RAN nodes, and RAN nodes hosting the AI/ML inference process. Examples of input information are UE location information, RAN energy efficiency metrics and RAN resource status measurements.
The identified AI/ML outputs consist of a variety of predicted metrics and actions. Examples could be predicted energy efficiency levels, predicted RAN resource status metrics and mobility decisions for the purpose of energy efficiency improvements or load balancing optimisation.
For each use case a set of feedback information was identified. This information indicates how the system performance is affected by the AI/ML based operations and it can be used, for example, to trigger model retraining. Feedback information is case-based and measured after AI/ML based decisions are taken. It may include, but it is not limited to, indications on QoS and UE performance, energy efficiency measurements, RAN resource status metrics.
See full details of the study in TR 37.817 – ‘Study on enhancement for data collection for NR and ENDC’.
What’s next?
The study on AI/ML support in 5G RAN concluded that, during normative phase:
- The working principles agreed during the study shall remain valid
- The functional framework should be used as a guideline
- The identified use cases should be the focus of the work and the solutions developed during the study should be taken as baseline
A Work Item based on the above conclusions was approved in March 2022 during RAN#95-e and is available in RP-220635.
Look out for progress on the new Work Item on Artificial Intelligence (AI)/Machine Learning (ML) for NG-RAN and consider joining us in our journey into the “uncharted” territory of AI/ML in NG-RAN.
https://www.3gpp.org/specifications-groups (select RAN3)
This article first appeared in Highlights, Issue 4.